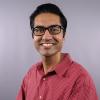
by Hammad Khan
by Hammad Khan
Many funding agencies today require data management plans (DMP) to be submitted in part of a research grant proposal. DMPs provide a roadmap for researchers in managing data. Funding agencies use DMPs for reassurance that the data will meet their sharing and openness policies. Institutions use DMPs to meet compliance requirements in preserving data for long-term use. To write up a successful DMP we must understand what is data management?
Data management is the process of managing data from its entry into the research data lifecycle to when it is finally archived into a data repository and made available for reuse. The research data lifecycle is a helpful framework to understand the different activities required in data management, those activities are planning, creating, processing, analyzing, preserving, and sharing. If the researcher knows what activity they need to do in each stage of the research data lifecycle process, it helps streamline data management activities.
In the planning stage one must think about the projects goal. What are the desired outputs, outcomes, and impact? What type of data is to be collected? Is the data numeric, text-based, or still images? Think about what format your data will be in. In this preparatory stage you are thinking ahead, so think about data collection procedures, software needed for analyzing the data, documentation in naming conventions and file versioning.
Data creation or what you may also refer to as the data collection and data entry stage. This stage is critical in that you must be consistent and accurate when you collect and enter data. In this process think about where the data is coming from? Is it Quantitative or Qualitative? How is it collected, through a research instrument or observation? What procedures for data entry?
The data processing stage is where you can prepare your data to be analyzed, which usually requires cleaning of your raw data. Remember to always save a copy of your raw data before you begin cleaning as you may want to come back to it later. When cleaning, monitor errors and validate accuracy, this can include minor spelling and syntax errors. Remember, in this process you are removing irrelevant entries and making sure the dataset is organized and ready to be imported into a data analysis software. Identify duplicate records and remove them. You may have to convert data from one format to another in order to be imported into your analysis software.
In the analysis stage you take your data and use data analytical tools, for example SAS, Python, and R to run statistical tests and try to find patterns and results in the data to answer your research questions. Think of this stage as extracting value from the data by applying data mining techniques or statistical analysis.
The next stage in the research data lifecycle is preservation of the dataset. Can you think of any research that may benefit from access to your data? Could your data address other research questions? Most likely the answer will be yes, which is one of the reasons we preserve datasets so that they may be of use in the future! Preservation is about ensuring access to data over time in a consistent and reliable form. This sometimes requires data curators to keep the dataset consistent and in formats in which it can be accessed. UTA’s institutional data repository, Mavs Dataverse, offers researchers a place to deposit their data for preservation.
The final stage is sharing your data through a data repository. Sharing data helps make your data available to other researchers. This is also when you may work with the data repository staff on creating metadata for your dataset and help organize and make consistent file formats and ReadMe file for the repository. Having quality metadata helps with reusability. Data repositories, like Mavs Dataverse, standardize citation of datasets to make it easier for researchers to publish their data and get credit as well as recognition for their work!
Good research data management makes your data Findable, Accessible, Interoperable, and Reusable (FAIR). FAIR principles are achieved through the practice of good data management. UTA Libraries provide Research Data Services (RDS) that can help you with data management planning and walk you through the different stages of the research data lifecycle.
Image Creator: Kukhyoung Kim. Copyright: Creative Commons Attribution 4.0 International (CC BY 4.0)
Add new comment